IEEE Region 10 Postgraduate Research Paper Contest 1st Prize Winner
Charissa Phua, MIEEE and Lau Bee Theng, SMIEEE, Swinburne University of Technology Sarawak
To avoid premature failures in the field, it is critical for wafers to be particle-free so that the final chip products do not incorporate any defects. However, at times, defects are generated during the lengthy and complex Metal-Oxide-Semiconductor (MOS) technology fabrication process.
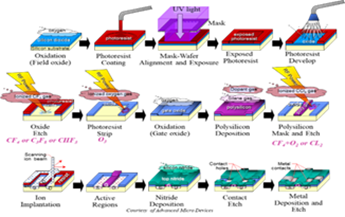
The rise of artificial intelligence (AI) technologies and the increasing demand for defect-free wafers encourage semiconductor manufacturers to pursue automatic defect classification (ADC). The current ADC system classifies wafer surface defects using optical and Scanning Electron Microscope (SEM) images. However, manual classification is still a major part of the process and it is not only labor-intensive and slow but also highly prone to human errors. In this research, deep-learning based ADC system is explored, which automatically classifies wafer surface defects, particularly from the metal layers. This approach brings consistency and speed, allowing for better determination of wafer life cycle as well as defect root cause analysis in yield management.
The proposed method adopts a deep convolutional neural network (CNN) architecture for defect classification using SEM images which can sub-classify defects into respective sizing groups whereby defect size serves as an important indicator of the origin of machine failure — the first in the field. This solution found that the proposed ADC method achieves industrially pragmatic defect classification performance based on experimentation with real semiconductor dataset. It is also apparent that the transfer learning for increasing the model’s training efficiency and training time reduction is worthy for an investigation.All the 2020 Region 10 Postgraduate Research Paper Contest winning papers will be invited for presentation at IEEE TENCON 2020 and the papers will be included in the conference proceedings.
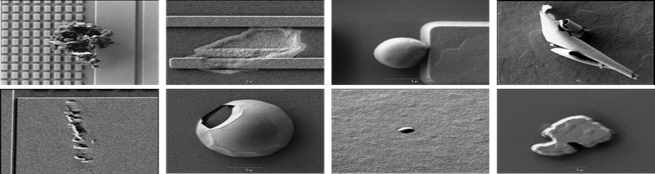